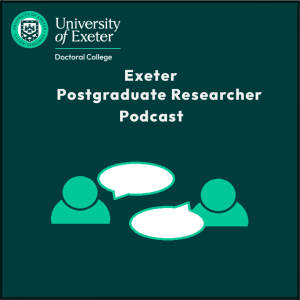
Monday Apr 15, 2024
Episode 3- Open Research (Professor Sabina Leonelli, Professor of Philosophy and History of Science)
Professor Sabina Leonelli (Professor of Philosophy and History of Science) talks to Dr Chris Tibbs, Research Data Officer at University of Exeter about open research, the use of Artificial Intelligence in research, and the importance of understanding the diversity of research environments when implementing open research practices.
Podcast transcript
Chris Tibbs:
Hello and welcome. I'm Dr Chris Tibbs and I'm the University Research Data Officer, part of the open research team based in the library here at the University of Exeter. My role involves providing support for researchers across the university as they work with and manage their research data, and today I have the pleasure to be joined by Professor Sabina Leonelli, a Professor of Philosophy and History of Science at the University of Exeter. So welcome, Sabina. Just to start, would you like to tell us a little bit about the research area that you work in?
Sabina Leonelli:
Thank you and hello everyone. So, I'm interested in the dynamics of research and research processes. Why is it that people who work in science use the methods that they use, handle data in particular ways, decide to publish in particular ways? Why do they choose certain research goals, and how does that occur historically but also conceptually? And what are the social implications of those choices?
Chris Tibbs:
That's very interesting. So you're really looking at these sort of different approaches and the different methodologies that different researchers are taking and that's very interesting because obviously different research areas will have different approaches and methodologies that they use. Now one thing that I noticed that you're very interested in, based on your web profile, is obviously open science and openness in research, and the European Commission and the United Nations, among others, all use this term of open science and just so that everyone listening is clear, open science is the approach to research based on openness and co-operative working, and it really emphasises the sharing of knowledge, results and the tools as widely as possible. But I just wanted to point out also that obviously these approaches can apply to all research disciplines, not just science. And so, for example, we are the open research team. And so, I tend to regard open research and open science as synonymous. So, I just wanted to get your take on this, Sabina. Do you see these as separate terms, or do you use them interchangeably?
Sabina Leonelli:
I also tend to use them interchangeably, but I think it is very unfortunate that it’s the term open science that has gotten so much mileage in the English language because in the English language we are aware of the fact that it does tend to be taken to refer to the natural sciences, more rarely to the social sciences, and never to the humanities and the arts. And this is different for lots of other languages. I mean, most, I guess famously the term wissenschaft in German tends to encompass all of the research domains, including humanities and the arts. I’m very partial to that, partly because I think that we're in a moment where research is so interdisciplinary and the boundaries between domains are so blurred, that actually making strict distinctions between what counts as a humanist approach, and what counts as a natural science approach, or a mathematical approach is becoming more and more difficult. As of course in history it has been very difficult throughout. So yeah, so I'm very partial to the use of the idea of open research in English, but of course we tend to use a lot the term open science too, because this is, as you were saying, very well recognised by policymakers and by funding bodies and a lot of people working in academia more generally.
Chris Tibbs:
OK. Well, thank you for explaining that. And again, the reason I just wanted to confirm this is because I want to ensure that everyone listening can be clear that what we mean by the term open science and that they don't feel that this doesn't apply to them, maybe because they don't see themselves as a scientist. So that's what I just wanted to clear up, and so that these practises do apply to all disciplines. Now moving on. Sabina, you hold many different roles and one in particular that I would like to mention is that you are the theme lead for the data governance, openness and ethics strand of the Exeter Institute for Data Science and Artificial Intelligence. So, given this particular role, I'd really be interested to hear your thoughts on how you feel artificial intelligence can play a role in the research process, and particularly around openness and the open research.
Sabina Leonelli:
Yes, thank you. So, I guess openness lies at the heart of what it means to do research, no matter how you look at it, right. I mean, doing research basically means trying to answer a certain question, trying to solve a problem that you may have encountered in your everyday life and within the more scientific landscape, it means doing it in a way that's a bit more systematic, that is susceptible to scrutiny and can be evaluated by others. So, research and science are public enterprises pretty much by definition. If it was only something that, you know, the one individual does in their own room, then it wouldn't really be something that we count as being research or science. And in that sense, openness, the availability of the outputs of research, being able to discuss the methods one uses, the procedure one uses and make them available for scrutiny really are what defines the very idea of research. So, given this, of course, the fact that we now have an emergence of more and more artificial intelligent tools that can be pretty much directly applied to the research process affects the ways in which we think about openness, because this means accelerating the research process to some extent. It means the potential to automate some parts of it, or at the very least work together with machines so that some of the, if you want, hopefully at least more tedious tasks associated to research, the more repetitive ones, the ones that more easily standardised can actually be delegated to machines and then can be iteratively dealt with in collaboration with humans. So in that respect there is a strong temptation to think that bringing AI into research processes will almost automatically improve the openness of research because it will allow, and in fact it will be almost incentive for people to make their methods ever more transparent, to make their data more available, and to be more careful in noting down the procedures that they’re using and make them available to others because all of those strategies make it easier to adapt research work to AI and to make it machine readable if you want so that machines can actually take over some parts of that work. The problem in assuming that AI automatically enhances openness comes at several levels, however. First of all, there is this problem, I'm sure many people have heard about of opacity in AI. The fact that because a lot of the reasoning that machines go through to produce certain outputs, so the type of algorithms that are used, particularly machine learning, tend to become less and less transparent and more and more opaque as time goes by – precisely because the machine is doing operations that humans wouldn't quite do or wouldn't be able to follow in the same way, and we can't quite track every single step that the machine is making in that sense. Then actually the system that is AI powered, becomes by definition less open because it's less obvious how do we read the system, how do we make it more transparent, more scrutinizable and more open for review. Given that there are all these parts of the system which are not necessarily intelligible to humans. So that's one issue that is happening in the area. Another very big issue is the fact that many of the providers of artificial intelligence technologies and particularly tools that are then applied in research, we can only think about large language modelling and tools like Chat GPT, which is produced by a company which contrary to his name, is not publicly funded but is a private company, Open AI. Many of those tools are privately funded. The ways in which they operate is even less transparent because a lot of the algorithms that are used, are actually trademarked, are not available for public scrutiny. A lot of the training data that is used to refine those algorithms is also not necessarily transparent, in some cases not even entirely clear that the data are data that are, you know, in fact right to use, they tend to have been data scraped off the internet in a variety of ways that may or may not be ethically acceptable. And so, we are in a situation where whenever we pick a tool from the internet thinking, oh, great, this is going to help me to do my bibliography; this is going to help me to write my essays; this is going to help me to search for, you know, literary sources on a particular topic, we are immediately given data and relying on tools that are not openly accessible, that have been developed in a way which is not immediately scrutinizable. And in fact, it may be using some of our own information in a way that is not open. So, let's just say that there are quite a few questions that are open around whether the use of AI in research in fact is favouring openness, and whether that's going to happen more and more in the future or in fact it’s going to have the opposite effect.
Chris Tibbs:
Wow, that's really interesting. I really love that sort of yes, you might think that it's going to help make research more open, but actually in fact it's not so clear and so that is really interesting and I think, the one thing I just wanted to add is about obviously any data that's being used by AI systems, the data need to be well maintained, well documented. You need good data going into training the models to help ensure that the outputs are accurate. And so, I just also wanted to mention that, as the saying goes, right, without data there's no AI. So, it's really reliant on having good data at that point. I just wanted to move on because I know that you obviously, like I said, you're working on many different projects, but one of your projects is a European Research Council-funded project called a Philosophy of Open Science for Diverse Research Environments, and given that title, I just wanted to hear a little bit more about this specific project and the aims of this project, particularly because obviously we've been talking about open science on this episode.
Sabina Leonelli:
Yes, thank you. So the project starts from the observation which comes from many years of me collaborating with scientists and studying lots of different research situations, especially in the domains of the life sciences and the biomedical sciences, and observing the fact that depending on what topics people are working on and what they're interested in, but also the specific locations they're working in, the materials that they're working in, they tend to work in very, very different ways and some forms of scientific research are highly dependent on cutting-edge technologies, for instance, others are not. If you're doing work in molecular biology, you may really want to have access to the latest model of genomic sequencer. If you are doing work on observational field studies, looking at how particular varieties of crops are growing in response to certain environmental conditions, you may in fact not be that reliant on that kind of technology and need other things. And similarly, you may have a situation where people are served by very good infrastructures. For instance, a very reliable broadband connection and reliable access to research facilities in situations where people don't have such reliable resources but maybe have other things going for them. For instance, they have access to very particular kinds of flora that other people in other parts of the world don't have access to. So there tends to be a huge variation in the conditions under which researchers can do very good research and produce really important knowledge. And this is not always something that is recognised by people who are writing about what it means to do research and what we mean by research methods, like indeed people in my discipline, the philosophy of science, but also policymakers, people who are thinking about the research landscape, how to fund it, how to support it. So, you know, funding bodies, for instance, the publishing industry, which tends to assume that people have a certain way of working and a more standardised approach to, say their experimental techniques or the kind of materials they maybe have access to, and this creates an issue when it comes to thinking about open science, because one of the interventions we're trying to do when we're trying to make science more open is to come up with guidelines and principles and policies that are going to push researchers and also especially research institutions to incentivise the openness of their work. So actually, put some effort into curating your data and making sure that other people can see what data you produced to back up your research, or put in some effort in looking at research outlets, which many people can access, and they're not just restricted to, you know, the very few people who have a subscription to a certain kind of journal, or making sure that when you're producing research, you're actually talking to people who may not just be academics, might be also be people who are interested in the kind of research and have some expertise in it, but they're not working in academia. So, all of those aspects are aspects of open science. But the ways in which they may manifest in research may be very, very different depending on the conditions on the ground. So, there is very often a tension between trying to produce some generalised guidelines that can provide these generally incentives for people to, for instance, share the research materials and the fact that when you look at different situations of different research domains and different locations, those vary same generalised guidelines may in fact prove to be problematic or sometimes downright damaging, right. For instance, very simply, when one thinks about sharing data, it looks on the surface like this great thing everybody should do it, it’s going to help research as a whole, but in fact it depends very much on who then is liable to pick up that data and do something with the data. If for instance, you are a researcher who's working on ethology, and so you are in the business of spotting, for instance, rare animal species and studying them and understanding their behaviour, particularly species which are at risk of extinction, then making all your data immediately public, including location data, so that you basically tell poachers where to find rare animals and how to locate them and potentially kill them all, may not be the most useful thing for you to do. Or in other situations, if you are the kind of researchers who's working with sensitive data, such as, for instance, most obviously human data, personal data, you have to be very, very careful about which of those datasets may be beneficial to share with other people and which not – may actually lead to unwanted implications. So, let's just say that the point of the project is trying to look at what happens at different locations of research. So, we're working with researchers in India, in Ghana, in Italy, in Greece, of course in the UK, in Germany, in Brazil and various other locations and looking at what they're doing on the ground, what are the challenges they are encountering and how they interpret the notion of openness in a way that actually would be helpful to them for the work that they're trying to do.
Chris Tibbs:
So it's very obviously clear that this one-size-fits-all approach just doesn't work across, as you mentioned, the different environments in which research is taking place. So, what can we do? How can we avoid this? What needs to be done to take into account those differences?
Sabina Leonelli:
Well, I think the answer comes from lots of different levels because there's so many people involved in the landscape and that's actually a good thing. It’s not just a responsibility of one agency or one person. So, at the level of research institutions and especially scholarly societies, I think it's very important that each domain of research tries to think very hard about what are the specific needs and the particular situations likely to emerge in relation to that research. So, working on phenotyping in plants, for instance, is going to be a very different requirements that people who are working on animal research in labs, or people who are working on clinical trials in biomedical research or in drug development in a synthetic environment. It's very important that there is an effort by researchers and the institutions within which they're working to think through what are the actual requirements for their field and how does one best think about openness in those situations. Then parallel to that, there should also be a strong effort to think about research as geographically dispersed. So rather than always think that, you know, the stereotype and the prototype. For best practise, say in molecular biology, is that particular lab in Oxford or at MIT or in Cambridge, so in very powerful, very well-resourced institutions which are sort of the very upper end of having access to a lot of resources. It'd be better to think a little bit more, you know, cogently about how do we adapt openness requirements to lots of, to the vast majority of situations where smaller institutions, institutions which are based in rural areas, whether that's in the UK or elsewhere, may not have access to all of those resources, and yet these are exactly the kind of institutions that researchers which should benefit the most from open science, at least in theory. What is happening at the moment is that because there's so little thought still about how do we get open science to be more just and to be more inclusive and to benefit really everybody who would like to participate. We have a situation where the biggest beneficiaries of open science activities are people working at institutions, which are powerful and have the resources to take advantage of things like big open data repositories and code sharing initiatives and things like that. While what we want is to actually lower the bar of entry into the open science ecosystem so that people who are working under very different conditions can also participate and benefit. And in that sense, this is an effort from institutions, from policy makers, also from researchers on the ground. Everybody of us can think about what are the best ways to make our research accessible to others, no matter the conditions under which they're working and whether, and that's of course a very controversial question, whether pursuing cutting-edge technology as the end point or the ideal goal for anything we're doing in research is actually always the right thing to do. Sometimes it is in fact better maybe to use kind of a low bandwidth tool to share our data or to go through a low-entry friendly interface, which may not be as sophisticated, but it's much more usable. For instance, doing coding or programming so that we can increase the usability and the inclusiveness of our tools, rather than always aiming for something which may be technically extremely sophisticated but ultimately may prove to be completely useless because very, very few people, aside from us can understand how this works and actually have the condition to use it.
Chris Tibbs:
Yeah, that sounds really amazing. If we can get to be that inclusive and really just take that extra time to think. Given your experience of working and collaborating with colleagues around the world, do you think that's something that's on researchers’ mind, like I mean, I guess not every researcher is even thinking about openness. So, I guess, are there even fewer researchers thinking about this and trying to be more inclusive? What's your feeling about how likely it is that something like this can actually happen?
Sabina Leonelli:
Well, I am cautiously optimistic about the research environment and research cultures because what I do encounter is a lot of people that may not be necessarily that aware of the open science movement or even that involved, but as soon as you start to discuss with them what are the challenges they see in their own work and what are the things that they would like to overcome, these kind of barriers immediately come up. So, I think a vast majority of researchers in my perception are well aware of the fact that there are very high entry points to participating in research in a variety of areas and that this affects negatively the quality of the research and the extent to which we can actually try and use research to address global challenges. So, in that sense, I think we are making progress. Where it's very complex is the fact that we are looking at a research system which is on the one hand very much subservient to a broader political economy, which is controlled by big tech companies, and this is important because all of us end up having to rely on things like Amazon Web Services and Google tools to carry out our research. And we know very well that this affects very much the choices that we're making in research in a way that we very often don't control. And so, the use of some of the proprietary tools really comes in the way of trying to implement openness to do it in a way which is more just, as we discussed. The other issue is that the incentives in academia overall, and certainly within Anglo American academia, continue to be not quite conducive to spending a lot of time thinking about what would be more sustainable, more responsible ways of sharing our research and making sure that we collaborate with others. Within UK institutions there is a lot of lip-service paid to try and co-design research and working, for instance, with local communities to try and devise solutions that make work for people. But when it comes to how researchers are evaluated, these are not the criteria that are used. We're still evaluated on the extent to which we published in very prestigious journals, impact factors, very often our citation numbers, the type of funding that we bring in, how much money we bring in, and these kind of metrics are very problematic when it comes to try and encourage a more intelligently open and justly open behaviour. So that's where I'm more pessimistic. I think we need to work very much on the system of incentives because otherwise, and also the platforming of the research, because otherwise, no matter how good the intentions are of people who are working, particularly in academic environment, they're still going to fall prey of this broader set of constraints.
Chris Tibbs:
Yeah, I think that's important. Researchers alone can't change it, right? The structures around them that are confining them also need to be changed. Like you said, needs to be incentivized to do this work. So, a little bit of optimism along with a little bit of pessimism. I just wanted to ask you one sort of a final question just before we finish up here. So, we talked about your research, you're doing a lot of really interesting research. We talked about the idea of openness, open science. We talked about artificial intelligence. So, to finish up, I would just like to open the floor to you. Do you have any sort of final take-away messages for our listeners today?
Sabina Leonelli:
So I think I want to talk specifically to early career researchers, PhD students, postdoctoral students, researchers, you know, beginning lecturers. I think it's such a fantastic time to be in the early stages of research or a research career. And I'm very aware of the fact that the job market is really not particularly good. But at the same time, there's lots of really interesting jobs in research also beyond academia. And there's so many opportunities to really think in a new and more creative way about what it means to do research at the moment. How do we use new technologies and how do we make sure that we make our research more open. I think some of the most interesting initiatives in terms of making more open research and more just research, more intelligent research, come from the younger generations as things like the reproducibilityTeas, you know, like younger people meeting and thinking, how do we improve the quality of research? What do we need to be able to do this? How do we avoid falling prey of commercial publishers? How do we manage to do research in a way that actually falls outside of these very well-defined traditional paths for having a career? I think all of these questions are very important and there is a real chance at the moment to try and change the system from the inside. I think there's lots of goodwill also by many senior academics who are themselves, I mean like myself and many other people, looking hard for answers to these questions and very much looking to the younger generation to inspire us as much as possible and to really try and let us know what they think should be done, given that these are the people that will carry out research in the future. So I would say very, very important to, even if you're in a system where you're under pressure in a variety of ways to try and do things in a conservative manner, to really use the impetus of being, you know, relatively newcomers on the block, seeing things in a different way, trust instincts into thinking if you see something that you don't think is quite right, that should be changed. Just pursue that idea. Very often you will find other people who are like minded and may in fact help you to try and change things from the inside. So, I really do think that a lot of impetus for change and transformation in research needs to come from researchers, and particularly the younger generation. And there are tools to do this. If you're interested, particularly in the question of data, data dissemination, data sharing, I would recommend that people look at the Research Data Alliance, which is a great organisation that brings together people from around the world to have these kinds of discussions. You will find people who are very like-minded and there is online working groups. They're very often in person conferences, so this is something they can really use directly. Certainly, the events organised by the Institute for Data Science and Artificial Intelligence very often will be conducive to having these kinds of discussions. We have a reproducibility network in the UK, which has a very strong base also in Exeter, and the people who are in charge of that would always be very available to talk to young researchers about what can be done. And also of course, you know, and Chris, you are one of the main people here, we have a wonderful library at the University of Exeter that can really help thinking these things through. So, I would just say don't get discouraged despite some of the obvious obstacles, seek out help and seek out alliances. And there's lots of work to be done in this area.
Chris Tibbs:
What a brilliant message to finish on. Thank you very much. It’s been really great to hear from you today. Thank you very much for taking the time, sharing your thoughts and insights, and really, really great. Thank you everyone for listening. Thank you, Sabina. Take care everyone.
Sabina Leonelli:
Thank you.
Comments (0)
To leave or reply to comments, please download free Podbean or
No Comments
To leave or reply to comments,
please download free Podbean App.