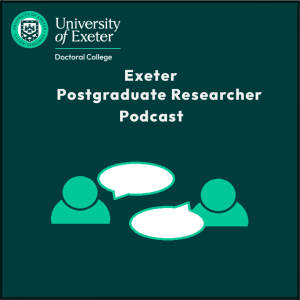
Thursday Nov 16, 2023
Episode 2- Open Research- Dr Gavin Buckingham (Associate Professor in Public Health and Sport Sciences)
Dr Gavin Buckingham (Associate Professor in Public Health and Sport Sciences) talks to Dr Chris Tibbs, Research Data Officer at University of Exeter about the different types of research data he works with and best practices for managing research data during your project.
Podcast transcript
Chris Tibbs:
Hello and welcome. I'm Dr Chris Tibbs and I'm the University of Research Data Officer, part of the open research team based in the library here at the University of Exeter. My role involves supporting researchers across the university as they work with and manage their research data, and so this episode is going to be all about research data and how best to look after it and manage it during your project. And to discuss all of this, today I have the pleasure to be joined by Dr Gavin Buckingham, an Associate Professor in Public Health and Sport Sciences here at the University of Exeter. So just to start with Gavin, would you like to tell us a little bit about your research and the different types of data that you work with?
Gavin Buckingham:
Hi, there, Chris. Yeah, I'm a cognitive psychologist by training, and I'm interested in human perception and human motor control. And I've been looking at this in the context of measuring the movements and forces people apply to pick objects up, and more recently I've been looking at this in the context of immersive virtual reality as well. Now, most of this data takes the form of pretty simple time streams, time series of data, so numbers representing forces or positions of things in multiple dimensions, and their expression over time. So many thousands of lines of data potentially that we then take maybe the largest value or the value at some critical other time points and that reflects some aspect of human behaviour. So that pretty simply is really what it is that we deal with here.
Chris Tibbs:
So thinking about all those types of data that that you're working with, I mean you mentioned, like numerical time series data. I just want to point out that, you know, data can also mean a wide variety of other types of data and many people might not think that they work with data. But generally, when I refer to data, you know, I'm thinking about any sort of information, evidence, materials that are being collected and used for that research. So I’d just like to hear your thoughts on, so when you're thinking about your data and why it's important that you look after your data and you manage your data in terms of helping your research and also then potentially making that data available.
Gavin Buckingham:
Yeah, it's a really interesting question because the pipeline that goes from the stuff that comes out of the apparatus that I used to capture people's data to the things that are subsequently reported in the paper, that's a pretty lengthy pipeline that has many different steps. And those steps can be fairly clearly articulated, but being able to show the consequences of each of those steps, I think is a really key part in terms of people being able to eventually understand your data and make sense of it and use it in other sorts of ways and I really feel that's the narrative I feel most passionately about in many ways. I'm perhaps, slightly selfishly, I'm not so interested in other people finding mistakes that are present in my data, God forbid, but I'm more interested in this resource that was collected that could potentially be a useful thing for other people in ways that I cannot even really imagine. That for me is the really big value I see in my dataset and I work with clinical populations. I work with children, with older adults, typically developing university aged people, all of whom have interesting ways that they interact with the world around them that you know could feed into hitherto unforeseen mechanisms or rehabilitation or technological advances and, you know, I really see sort of the value of data just sitting there waiting for someone to be able to harvest in that way.
Chris Tibbs:
Yeah, all of this sort of potential that's in that data, that you know, doing analysis that are just completely irrelevant, that are completely separate from your research. So when did you sort of first start thinking about making, like managing your data, to make it available so that others could have it, and be able to analyze it? Was this sort of something that you had a discussion with, maybe your supervisor as a PhD student? Was this something that, you know, you sort of just picked up on sort of later during your career?
Gavin Buckingham:
Yeah. When I was a PhD student and postdoc, this wasn't really part of the narrative at all. There was no real sense that this is what you would do, but it was actually more to do with the experimental and analytical code: the MATLAB files in my case that I fairly vividly remember asking someone if I could use the MATLAB files to run an experiment of my own, and they're like, well, these were developed in collaboration with my colleagues and it cost money to get these developed, so probably not. And I was sort of thinking to myself, that's a bit of a disappointing perspective given that this doesn't directly earn anyone any money and gate keeping it from me isn't stopping you getting the benefit from them. So when I got my first lectureship, I was given, as part of my start-up contract, a research system to help develop the code that would underpin the data collection in my lab and I was sort of very clear in my head that data will be available to everyone and I started creating a wiki from my lab webpage and you know a lot of this is lucky me to have the resources and the skilled person available to do this and set this up from the beginning. But really that was kind of the key, the key step as far as I was concerned. You know, once all of this MATLAB code to control the data acquisition unit in the force transducers that underpinned all of my research at the time was up online. That was number one, a really nice way for me to stay on top of something that someone else had written for me, which was a new experience for me anyway. But also to share with the world and you know, I mean sort of going forward since then I've had seven or eight people set up their labs with that code and it's a pretty niche research field, but it feels really nice to know that that code has been used in this way for this particular purpose. And then from then the sharing of data kind of felt like a pretty natural step once that became part of the narrative on social media in particular, is seeing people talk about this on Twitter, that has been really formative part of my education in this area.
Chris Tibbs:
That's really interesting and just picking up on something. So you mentioned this wiki for your lab. So, this is obviously something that that you discussed with your team and with the PhD students that you supervise. So I mean, you made a concerted effort that this would be part of this. Obviously, they're learning when you're helping them to develop as researchers on their own. You made a concerted effort that this would be part of that process?
Gavin Buckingham:
Yes, although maybe perhaps not as aggressively as one might imagine. I certainly don't mandate things like data sharing or sharing of code, because at the end of the day, particularly if you're future life is not likely to be outside of academia and you have potential intellectual property issues, or you want to display your own evidence of your expertise, that's done in very different ways in very different fields. So I encourage and I support my trainees to provide basically everything as open as it possibly could be, but I'm not that interested in mandating it to them. As it stands, they've been even more enthusiastic in their uptake of this than I have and you know, certainly some of my PhD students have improved my own nascent processes quite substantially and taught me things and do stuff a lot better than I'm able to do as it stands.
Chris Tibbs:
So do you have any tools or techniques that you could share in terms of, so some of these examples of where you and students from your lab are sort of building-in these sort of best practices? You mentioned the wiki, you mentioned about data sharing. So is there any, you know, like sort of examples of like a tool or you know something that you could sort of just share, some sort of, this is one thing that we have done in our lab?
Gavin Buckingham:
Every project that gets up and running in my lab, there's an Open Science Framework (OSF) page created for it. That Open Science Framework page might exist as nothing other than a place to put a preprint of the paper at the point of publication. So I know that everyone has access to at least the version of the scientific outputs, which I feel very, very strongly about. That seems like a complete no brainer, zero effort thing to happen. Oftentimes that's accompanied by a pre-registration document, be it a version of the introduction that we'd sort of hashed out together, me and the trainee, or a template from As Predicted or something like that. Eventually, this is also often populated with individual participant data and then the summary statistics that would have been used to calculate the F ratios and P values and things like that, and the statistical analysis and the supplementary materials that would go alongside the paper as well. So it becomes just this wonderful, convenient storage place to segregate everything to do with that particular research project, which as I've progressed through my career and I am working concurrently on what feels like 1000 different things at the same time, it's incredibly, I would say essential. An essential part of my practice, because otherwise I'd be like relying on my, uh, incoherent filing system to keep track of everything, whereas now I can look in my OSF page and all the things that are shared with me and capture a huge amount of stuff that's actually really useful for me.
Chris Tibbs:
Yeah, that's really interesting, that’s a really good way to manage it. So I just wanted to highlight a few of the points that you raised there. So like having all of the documentation alongside the data, right, because it's obviously important, the data by themselves are essentially meaningless. So having all that documentation alongside the data is obviously important and having the data available, so alongside the publication, when someone reads the publication they can obviously access and see the data. I also, I just want to mention, so you obviously talked about depositing the data and the documentation all in the Open Science Framework which again is totally fine. I just want to, obviously highlight, point out, that the University also has a repository that can be used not quite in the same way that you use the OSF. The repository, Open Research Exeter is more for the published dataset to go alongside the publication. So just talking about publications, so you talked about preprints, you talked about you know, pre-registrations, registered reports. I just wondered if you could say a little bit more about particularly the pre-registration and registered reports as these are sort of new methods of publishing and sort of what it is they're trying to achieve that’s sort of maybe different from a standard publishing process.
Gavin Buckingham:
Well, it's interesting that you sort of call it a new narrative, and it's definitely a new narrative, but one of the things that drove me in this direction was when I moved to Exeter, actually, I moved to a department that has this incredibly onerous ethics process. An ethics form that's some 20 pages long. And this for many disciplines seems like a completely bizarre idea, but it actually forces you to directly confront the background, the things you're hoping to measure, the things you're hoping to manipulate, and why you're hoping to do those things. And articulate who you're going to recruit and why that sample size, complete with a power calculation. So all of this stuff needs to happen before I can start collecting data, whereas back when I was a postdoc, I would apply for ethics with a fairly simple, this is what I'm going to do - it's pretty safe, so that will be fine. Here it's a much more onerous process, but this actually means that I already know all of this stuff at the beginning. So creating a pre-registration, where I have articulated what it is I plan to do, what it is that I hope to get out of this, what I'm going to do in terms of statistical analysis and even deeper details like how will I deal with outliers and what sort of things will I have in place to, you know, foreshadowing all those difficult research decisions that I might have to make later on that I've sort of forgotten I will have to make later on in many cases, is a really useful thing and it was sort of happenstance, really, that this ethics process landed at just the right time at which pre-registration opportunities were billowing into the, certainly the psychology ecosystem, through things like As Predicted, through Open Science Framework growing up, and as you say, through registered reports, which are a version of pre-registration where your study protocols are peer reviewed before you collect the data. And this in many ways is almost like, you know, how it is with a student and the supervisor. They come to you, they pitch an idea and then you refine it together and then you're finally ready to start off. Here, it's not just you and the student in your you know, little bubble, it's you and some reviewers who have really crafted what's the perfect experiment to answer this question. And then you go out and collect the data safe in the knowledge that no matter how it pans out and it's a significant difference, no significant difference, slightly awkward P value that sits in the middle of being able to be interpreted as one thing or the other. The publication will still happen and it will be accepted assuming that you stick to what it is you said you were going to stick to. And then, you know, you still have the opportunity to explore your data and in the way that you would have, uh, before the days of registered reports and pre-registration anyway. But it's a sort of really interesting publishing pathway, although one that I probably haven't embraced quite as fully as I would like to. I've done one registered report myself to date and hopefully there will be more, but I think that the challenges of identifying exactly what you're going to do to a dataset before you collect it are not ones that are easily overlooked. I think that you need to be very certain about the protocols and exactly how this data looks. It needs to be really firmly in your wheelhouse of expertise. Can't be a sort of study that's branching off into a slightly new area using a new technique, using a new data collection method. I think it's really got to be something that you know a lot about and for that kind of study to pop up just at the time when you're maybe a bit later in your career like I am, you know, it's a reasonably rare occurrence. That said, I think if not for the pandemic and if not for the things that had done to various data collection timelines and uncertainties thrown in there, I would like to think that many of my PhD students would have submitted stage one registered reports by now and be collecting data for them. It just really didn't seem like a pragmatic thing to do back in 2020.
Chris Tibbs:
Yeah, I just, I mean that's really interesting that these, you know, sometimes these things just align, they just align up and things just work out. Something else I just wanted to mention as well was because I mean, you talked about this, right? So this, you know, your sort of move into this sort of you know, Open Research area wasn't something that you sort of developed as a PhD student or a postdoc, right? It's something that came sort of a little bit later, and I think that's important because I feel like this is sort of an example of it's not too late to learn, right? So just because maybe you're already a lecturer, you're already well established, doesn't mean there's not things that you could still learn or things that you could still implement. So I think it's important just that we can say that this is like, is not just something that, you know, you want to pick up as a PhD student, I think. You're never too late to learn, as the saying goes. You've obviously been, as I mentioned on this journey regarding looking after, managing data, sharing data. I just wondered if you could maybe, you know, highlight maybe some of the obstacles that are, you know potentially in the way that you think we as a community might still need to address.
Gavin Buckingham:
Yeah, I mean, the obstacles are plentiful. Two that sort of spring to my mind initially are what do we do with the old data, as in the sense of currently my workflow will be to have our participants consent to having their data shared in an open repository. But again, that's something that has, come in over recent years, what about people who did not consent to that either because I never asked them or just because no one ever thought to ask them back ten years ago? Should that data go up online? Is that fair game to go up online? What has GDPR done to that? And what are the interpretations and legal consequences and how do they vary from institution to institution or data protection officer to data protection officer? And I really feel that these challenges are often so overwhelmingly insurmountable that many academics will just go probably best for me just not to bother, and I'm pretty sympathetic to that idea. I certainly at one point had all of my data up online and then I decided, probably based on watching something on Twitter, maybe I should just put the data that people have explicitly consented to up online and you know that's a sort of awkward position to find yourself in any way as an academic. I think the other big issue that we get to confront here is what is raw data. And the least raw data or the lowest barrier to entry, I think is let's put up the CSV file or the SPSS file or the R file that contains your summary statistics. The average of each person in each condition. Maybe a 20 by 30 matrix in my sort of a typical case, and then someone can do the same statistics I did taking my word for it that those numbers are real. Fine in some sense, useful for maybe doing a meta analysis and being able to calculate things I didn't report in my paper, such as confidence intervals or things like that. But less useful in other contexts, and certainly not raw data that you could learn anything about human behaviour from. Really, it's data presented in the way to answer the question that I wanted to answer. I could present my rawest of raw data: what comes out of my motion capture cameras? That's just about achievable for me, but each of those files is several megabytes and you know in a large study with many trials that quickly turns into gigabytes. If you're in bigger data worlds than I am, that becomes unfathomably large, in which case you need to start to rely on the university structures. Which isn't a bad thing. It's nice that the university structures have kind of caught up with this potential demand, although I suspect they're not utilized nearly as effectively as many people behind the scenes would appreciate them being utilized. Or the rawest data are completely identifiable to participants and we break away this anonymization. Thinking of an MRI scan of someone's brain, for example, quite easy to determine whose brain that is, or, you know, once you have the key to unlock that piece of information. In the world of movement control, it's still a little bit up in the air. You would probably assume that the way someone moves their arm to reach out and pick something up is not at all identifiable to that person, but with good enough mathematics, and particularly in the world of virtual reality and data sharing, which is a very hot topic around the main company that's involved in immersive virtual reality these days, Meta. There's a lot of unanswered questions that leave a lot of uncertainty, and it's definitely easier to err on the side of caution I feel, whether from a legal perspective or from an ethical perspective, and finding that right balance is definitely a study by study situation by situation challenge that makes it very hard to standardize processes and protocols without me ultimately having to make a judgment call.
Chris Tibbs:
Yeah, I think that's very important. It's very, it's not nice, straightforward here's the data, every time. It's complex, right? And more so when you're dealing with human participants, and you have the ethical side of that. So yeah, it's not straightforward. And all of this takes time, obviously. All of this takes, you know, resource that, you know, that someone, the researcher usually, has to do that work, right? And so it's, yeah, it's complicated and there's no, at the minute, I don't think there's a straightforward answer or you know, one size fits all solution to that. So if, you know, we discussed various different things today. So if someone was listening and you know they were thinking about you know this, this sounds like it should be sort of the approach that I'm taking to my research. You know, I should be looking after my data, should be sharing it where possible. Do you maybe have like a sort of simple take home message for that listener? So maybe they're feeling a little, you know, overwhelmed. Not sure where to start. Would you sort of, you know, have maybe one simple message for how they could get started?
Gavin Buckingham:
Yeah, and that simple message is that doing everything or something a little bit better is better than it was beforehand. And it can seem like the barrier to entry of the open science world and the reproducibility world is so high and you need to pass so many purity tests to be able to feel like you're one of the gang. That's definitely a narrative that I have no interest in. That's, I'm not some superstar of the open science and reproducibility world, I'm just a normal academic who has little bit by little bit been able to make fairly incremental changes that have, I think, substantially improved the way that my practices are from when I was a junior academic and you know this could be as just as simple as uploading all your papers on to your website so that they're all not just available, but you know, easily searchable, that's open in some senses, putting up the code you use to collect your data or analyze your data with the idea that, well, maybe someone else will be able to use this and save them a bunch of time and you're contributing to science that way. And I would say these things ultimately are hugely important aspects that will seem like everyday working practices for some people, and then you can think to yourself, well, actually, yeah, OK, well, I do these things already. Maybe my next study, this one seems like it will be appropriate for data sharing and maybe I'll do a bit of a pre-registration for this document for this next study because well, you know it's probably going to be useful for me. It will certainly save me having to try to re-remember why we did this and what we said we were going to do to remove the outliers and stuff like that back when we had this discussion a year ago. And then from there it becomes almost a fairly natural thing to think, well, let's just do a registered report. The timelines sit perfectly for this I'd like to try something new. It's kind of interesting to shake up what feels like a slightly jaded publishing process by the time you've reached my stage in my career, and it was actually a really refreshing feeling to do something new and different. And it wasn't at all any more or less effort than a traditional publication process, but it was quite a lot more fun I felt than the typical workflow I go through and you know, I think the opportunity to shake these things up is one to be grasped. That so not really a short answer, but there we go.
Chris Tibbs:
Well that’s really good advice. I really like that, you know, take small steps, right, small steps can lead to big improvements. I think that's really good advice to end on. So Gavin, it is been really interesting to hear from you today. Thank you very, very much for sharing your knowledge, your experience and hopefully maybe we can inspire some listeners to start taking that first step. So thank you very much Gavin. Thank you very much everyone for listening. Thank you.
Comments (0)
To leave or reply to comments, please download free Podbean or
No Comments
To leave or reply to comments,
please download free Podbean App.