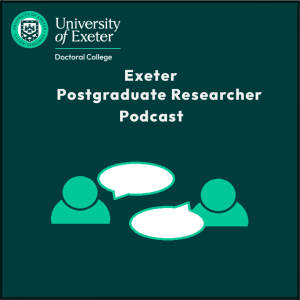
Tuesday Aug 15, 2023
Episode 1- Open Research- Dr Eilis Hannon (Senior Research Fellow in the Complex Disease Epigenetics Group at the University of Exeter Medical School)
Dr Chris Tibbs, Research Data Officer at University of Exeter, discusses research data and how best to manage that data during your project with Dr Eilis Hannon, Senior Research Fellow in the Complex Disease Epigenetics Group at the University of Exeter Medical School.
Podcast transcript
Chris Tibbs:
Hello and welcome. I'm Dr Chris Tibbs and I'm the University of Research Data Officer, part of the
Open Research team based in the library here at the University of Exeter. So my role involves
supporting researchers across the university as they work with and manage their research data, and
so this episode is going to be all about research data and how best to manage that data during your
project. And to discuss all of this today, I have the pleasure to be joined by Dr Eilis Hannon, a senior
research fellow in Clinical and Biomedical Sciences here at the University of Exeter. So Eilis, would
you like to tell us a little bit about your research, what it involves and the different types of data that
you work with?
Eilis Hannon:
Yes. Well, thank you very much for inviting me along today. So I'm based in the complex disease
epigenetics group and we have a group of mixed modalities. We've got wet lab scientists and dry lab
scientists, like myself. So we generate and analyze quite a lot of genomic data. So we're primarily
interested in the brain and modelling gene regulation in the brain and we're in a really exciting time
where there are so many different technologies and experiments that we can take advantage of,
that the quantity of data we've started to generate has just kind of exploded. So from one single
sample, we can have kind of, you know, be 4, 5, 6 different experiments and kind of layers of data.
And so what I'm quite interested in doing is trying to integrate those different layers together. So a
lot of what I'm working with is experimental data, but because a lot of these technologies are quite
new, we're often developing new methods to analyze them in parallel. And so what we also do
sometimes is simulate data where we kind of know what it looks like. We know what the outcome
should be to kind of test and develop methods. So it's quite a broad spectrum of different data type.
Chris Tibbs:
Yeah. So you mentioned it there, right? So you maybe have simulated data, you've experimental
data, and so I just wanted to pick up on the point here when we're talking about data and this
obviously might mean different things to different people. And so if you're listening to this
discussion and thinking, oh well, I don't work with data or this doesn't apply to me, then I just want
to really make clear that when I refer to data or research data, it really means all of the information
or the evidence or the materials that are generated or collected or being used for the research, and
so that we're clear about data and what it refers to. Why is it so important to manage this data
effectively? I mean, you talked about you're producing a large quantity of data, so I'm guessing that's
one of the reasons why it's important to look after it.
Eilis Hannon:
Yes. So from my point of view, efficiency in terms of processing that data in, I mean you know if it
wasn't organised in a kind of sensible or a kind of pre-planned format, then it would be incredibly
challenging to work with, so from you know, we take advantage of the high performance computing
available at the University and so to do that efficiently, we need to kind of have some pre-described
format for the data. But there's also ethical implications. So, you know, we're working on data
generated ultimately from a piece of human tissue. So we have requirements in terms of how we
look after that data, what we do with it. Who uses it and how? So we need to make sure that you
know our data is organized that such that those requirements can be met. But also, you know, one
of the really nice things about what we do is from one experiment you can answer lots and lots of
different research questions. So different people within the research group will be taking advantage
of the same dataset. And to, you know, to really maximize that utility, we need to, you know,
organize it in a way that we can find it. We know what's what. And we can really reap the benefit of
that initial kind of financial investment.
Chris Tibbs:
Yeah. So it's obviously clear, especially if multiple people are working, doing different analysis on the
same data. It's obviously important to know what the data are and make sure that they're obviously
described and who's doing what on the data, and version control, I imagine is something that's very
important for you. Like, it's clear that the data are fundamental for the research, right, and it doesn't
matter if you have, you know, the most sophisticated methodology to analyse the data, if the data
are not described or the data are inaccurate then your results are not going to be good. They’re
going to be inaccurate. They’re not going to be clear. So this is something obviously that you're
doing at the minute, your managing your data. When did you really first start thinking about the idea
of, you know, managing your data, particularly with the aim of potentially making it available to
others to validate or to build upon your research? Was this something that your supervisor discussed
with you as a PhD student or was this something that you sort of picked up later on in your career?
Eilis Hannon:
So during my PhD, which I did in Cardiff, I was using publicly available data and so I had quite a naïve,
I guess, view of kind of experimental work and when I came to Exeter and joined a team where we
generated the data we analyzed, you suddenly start to realize that, you know, of course,
experiments aren't perfect. Of course they don't work as expected all the time. And you know, I
gained a real insight at that point because obviously questions about how we use the data, how we
process the data and how we ultimately share the data became a lot more relevant to my work. But I
also gained a huge insight, you know, being much more aware of the whole kind of research process
from kind of study design, generating data, analysing the data and publishing it, you know, kind of
what the requirements were and also the kind of challenges with data generation, and so that was,
you know, I strongly recommend it to anybody who sees themself more as an analyst, that actually
the insights you gain from working closely with the people that generate the data are just
unfathomable really. It really opens your eyes and gives you a much, I guess I think much more
holistic view of research.
Chris Tibbs:
It's really interesting how your perspective changed from someone who's just analyzing the data to
someone who actually is experimenting and generating the data, right? That's a really interesting
view. When you start to be someone who's producing data and potentially sharing it, then it's a lot
more important to think about all of these processes. So talking about these processes of looking
after the data, I mean what sort of tools or techniques would you recommend to someone who's
interested in, you know, making sure that they manage their data effectively or looking after their
data?
Eilis Hannon:
So I think, forward planning where you can. Thinking about where you're ultimately trying to get to
in terms of you know what format do you need the data in to do the analysis that you want to do?
But also thinking about kind of, particularly working with large datasets like we tend to, we can't
store kind of multiple iterations. We need to be quite practical about what are the core stages that
we need to stay, and actually if you sit down and think about it, for us the most important parts of
the data are the raw data and then our analysis scripts, because from there we can recreate
anything that we've kind of done after that point, if we were to lose it in some kind of, you know
freak event or something. It's very tempting to hoard these kind of intermediate datasets, but often
they actually make your life much harder because you can't actually remember at what stage each
file relates to. And so the kind of more streamlined you can be, in terms of what you save and what
you keep, does actually make management during these data much easier and, you know, clear
records in terms of having scripts can also help you navigate that process. And as you become kind
of more ingrained in your project, you do start to realize what the kind of critical points are that you
want to kind of save and keep a record of.
Chris Tibbs:
So you mentioned two points there that I'd like to pick up on. First of all forward planning, which I
completely agree is very important. And so I just wanted to at this point highlight sort of the
importance of a data management plan to do exactly that. And so this is the plan that you develop
sort of at the beginning of the project and thinking about all of those things that you talked about in
terms of what it is you want to do with the data and trying to think about them from the beginning
so that you can identify potential obstacles or issues and then try and plan around them to mitigate
them. So that's definitely very important. And then the other thing you picked up on was about code
and reproducibility. So would you, would you say that for someone who's, you know, working in a
similar area or with similar types of data that it's really a requirement to learn a programming
language, so Python or R, to really ensure that not only is their analysis reproducible for someone
else, but also for them. So like you mentioned, then you just need essentially the raw data and the
code, and you can reproduce the analysis. So would you say that’s sort of a requirement?
Eilis Hannon:
I would strongly recommend it, purely because of what you learn alongside a programming
language, and that is, things like how to record what you've done. So it is in my opinion, it's the most
transparent method section you could ever write. It tells you a lot more about what you actually did
than someone could ever gain from reading a paragraph where you describe what you did. And it's
also really, you know, if you do it in a way that it automates your analysis such that you can know
confidently that every line in this script was run from top to bottom. It's really easy then to backtrack
if you find an inconsistency further down the line and really easy to make a change and re-run it. You
know it's frustrating, of course, and something you can spend a lot of time focusing on the oh all that
time I wasted because of that error. But the beauty is that you can fix it really easily and if you can
just let go of the kind of regret, that problem is fixed in a way that you could never fix it in the same
way from the actual experiment. So we do have the ability to repeat our analysis. We do have the
ability to make tweaks and we should kind of embrace that rather than see it as the kind of aww but
all that time I wasted, you know on the incorrect version and see it for the positive that it can be.
Chris Tibbs:
Yeah, the only thing I can add is document your code. It is so nice when you go back to an old code
and you see that you've documented it so you know exactly what each of those steps were and why
they're there. So yeah, definitely. Related to the... Oh, sorry, did you want to say something?
Eilis Hannon:
I was just gonna say that that I'm gonna echo, I think it comes from the software carpentries and it's,
you know, the person that you're gonna benefit the most is your future self.
Chris Tibbs:
Indeed, and related to code, so you were awarded a Software Sustainability Institute (SSI)
Fellowship, which is about, you know, providing funding to fellows who are trying to improve
research software in their research areas. So would you like to say a little bit about that experience
and also just about the work that the SSI are doing in general?
Eilis Hannon:
Yes. So the Software Sustainability Institute kind of comes from recognizing that research is highly
dependent on software and often highly dependent on bespoke software or computer programming
solutions. But often, these aren't easy to reproduce, or even to find and the sharing of this software
isn't brilliant really. And that's partly because the kind of emphasis on research is writing the paper
and extracting the results from the software, rather than thinking about the software as a research
output of its own. And so the Software Sustainability’s kind of mission is to just improve the quality
of research software. So it does that by funding fellows at all career stages in all disciplines to kind of
support the development, maybe by running workshops, or petitioning their local institution or
funding bodies to try their best to recognize these research outputs. So one of the kind of parallel
initiatives that we have, a research software engineering campaign that's kind of at least 10 years
old now and in the last two years Exeter have a central group of well of about 10 members and you
know that's again recognising that within a university ecosystem we need people whose expertise is
programming and can help us process our data, maybe do new things that we never thought
possible because of the skills that we now have available. Yeah.
Chris Tibbs:
Indeed, yeah. Indeed. It's very important to have those skills and have those skills recognized. So a
big part of what we're talking about here in terms of managing data is also about the idea of sharing
that data, where possible, so that like we talked about that others can come along and they can
validate and replicate that. So I just wanted to ask you about your own experience of sharing data,
you know, putting data in a repository or you know data associated with the publication and how
you make sure that the data and the publication are both seen together and how users or readers of
the publication can access the data. So have you deposited data in a repository? Is this something
that you've done?
Eilis Hannon:
Yes. So we, routinely deposit as much of our data that we can often because you know we're funded
by the Medical Research Council and it is a condition of the funding that we share the data that we
generated. Occasionally you know we're working in a study design where the kind of ethics don't
permit it, but we try to find, you know, an alternative way of giving people access to the data if they
need it. So maybe by some kind of application process. The route we typically use is to deposit it in a
public repository, so we use the Gene Expression Omnibus, which is hosted on the NCBI website and
the first time we did it, I'll admit it was a little bit of a faff because they have a fairly rigid format,
unsurprisingly, and the only reason it was a faff was because it was something we thought about at
the end of the project as opposed to the beginning of the project. And so we hadn't necessarily
organized our files in the way they wanted them, so we kind of had to go back and re-extract some
of the raw data. But once you’ve done it once, the format typically stays the same and so next time
round we were able to better kind of plan ahead for that. And so it's a more efficient process for us
and actually one thing we keep, because these are public repositories, we often think that there's a
huge advantage for us to deposit our data actually at the beginning of the project because it's
another way of backing it up. You know, we don't have to spend so much money ourselves on data
storage because we've put it on someone else's server and you know, not only do other people
benefit from being able to download it, but we can redownload it if we need to in the future. And
also caveat that even though we might deposit it at the beginning of the project, you can put it
under an embargo so it's not publicly released until you kind of say yes, go ahead and do that. So it
can be quite a useful you know, we put an embargo on for maybe a year. So you know we can still
do our analysis, we can still, you know, write our paper up and then typically at the point of
publication is when we would say open it up to share it with the wider world.
Chris Tibbs:
Yeah, it's really interesting and thinking about the benefits of sharing it at the beginning that then
you don't have to look after, you know exactly where it is. So I just wanted to pick up on a couple of
points. You mentioned, obviously you depositing your data with the Gene Expression Omnibus,
which obviously specializes in genomics data. And I just want to point out obviously that the
University has an institutional repository, Open Research Exeter or ORE, and although we have a
repository, we do recommend that data should be deposited into specialist repositories where they
exist. Obviously if the specialist repositories don't exist then that's where ORE comes in. You can
deposit your data into ORE. And something else that is also very important is, you know depositing
data in a repository is important and making it available to others. But it's not enough to simply
make the data available, right. You need to make sure that others can understand the data and so
this comes back to then, making sure that the documentation explaining the data are also available
alongside the data. And then in terms of publications. So often you've got data underlying a
publication. When you deposit your data into a repository then the data are often assigned a
persistent identifier and this identifier which obviously uniquely identifies that dataset should be
included in the publication as a way of making sure that someone reads your publication and thinks,
oh that's really interesting, I wonder if I could use that in my own research and they can click on a
link and go and find your data and then use your data and build on it. And so it's all about making
the links and making everything clear so we know that others know exactly what the data are and
therefore how they could potentially use them. So you talked about with the SSI trying to see
software as this important research output, and I also feel the same way about data, right? It should
also sort of be seen as this standalone output that's very important. So I just want to, I assume you
have similar thoughts to what you do about the software in terms of having data as a standalone
sort of output.
Eilis Hannon:
Oh, definitely. I mean, so when we kind of, you know, when we receive a grant from say, the Medical
Research Council, they're not only funding us to answer the question we outline, they are funding us
to generate a dataset and they want us to, you know, they see the value of the dataset as a research
output. And you know, that's why they kind of require that we share that and you know, certainly
that's one of the selling points that we write into our grants is that you know whilst you know we're
generating it to answer this question we recognize there is value more broadly and we want to help
facilitate that. You know and I think that's one of, I'd say that's one of almost the misjustice is
probably too strong a word, but actually there is a lot of pre-existing genomic data. So if I talk
specifically about my field, that could be reused a lot more to answer a lot more questions, yet
arguably the emphasis is still on generating new datasets and there's a little bit of a gap where you
could fund analysts or computer scientists to come in and take advantage of publicly available data.
And of course, that's actually quite a cheap grant, because you just paying someone's time as
opposed to paying expensive experiments. But I don't know that the research landscape’s
necessarily picked up that actually we could. You know, there's probably a lot of answers that we
could make if we just funded a few more analysts in the field as opposed to constantly funding new
data generation projects.
Chris Tibbs:
Yeah, that seems like it’s missing a trick there with all the information and results that could still be
generated from those archival data. I just wanted to perhaps ask you someone obviously who's sort
of been on this journey from analysing publicly available data to then going and generating your own
data about some of the obstacles and barriers that you have found and maybe potentially found a
way around.
Eilis Hannon:
So I think one of the biggest obstacles, I'm gonna talk specifically about code sharing code here.
Chris Tibbs:
OK.
Eilis Hannon:
And because that's, I guess, a big a kind of focus of mine and I recognize one of the barriers is I guess
a feeling that my code isn't good enough to be shared. You know, it's not pretty enough or it's a bit,
you know, I'm not an expert programmer, you know, I've taught myself or I've only been on a
beginner’s course. And I'm kind of only here because I've had to get here and therefore I'm either
exempt from sharing my code or, you know, generally there's a lot of anxiety that it doesn't look nice
or, you know, someone might find a mistake. And you know that, a PhD can be a time of, you know,
fear that, you know, there's so much pressure on my results and you know if someone were to find a
mistake and that could be quite, have a big impact, but I'd actually flip it around and say that if you
approach your project with the intention of making it open, you tend to program in a different way,
and you tend to program in a more reproducible, robust way and actually think of that almost as,
rather than thinking about people finding the mistakes, think about the fact that what I have been
completely transparent in what I did. OK, maybe I haven't done it. Maybe somebody would disagree
with the way I did it, but because I made my code available, they can see exactly how I did it and
under what limitations that is, and my results are what they are based on this, you know, based on
these decisions that I made and I have to say that the main benefit I've seen is my own personal
satisfaction in my work. Because, you know, yeah, OK, there's lots of different ways we could have
done it and somebody might criticize the way I did it, but they know the way I did it. And so they
know the extent of my results and maybe they would’ve done it differently. But, you know, I've been
transparent and how I did it. So they know how to interpret what I did and it really helps remove
that anxiety about someone finding a mistake. If you think about it, it's just you being open in how
you did it such that somebody can assess its value or not.
Chris Tibbs:
Yes, very important about this all about having the mindset of approaching it from being open from
the start. That's really interesting. So I just want to wrap up by asking one final question. You know, I
mean you sort of touched on this in the last answer, about having this mindset of having, sort of,
open from the start, but given your experience, like is there sort of one simple take away message
for listeners who, they want to do the right thing, they want to look after their data, they want to be
able to make it available, but they might be feeling a little daunted and not really sure where to
start.
Eilis Hannon:
So I think. There are lots of small steps that can be taken, and so if you're going, you know, if you're
new to this, then you know the first thing might be the first step would just be to have a script that
you know works and it's nicely commented and documented. Yeah, that's the first natural step. You
know, and I think that actually this isn't something that you have to kind of go into expecting to be
perfect from the start. There are lots of little things that you can do that just get you towards a more
open environment and the one thing we have to be conscious of is that the requirements for
different types of research vary hugely, so there is no one fits all solution here. It's all about what's
relevant to your specific project and you know the outcomes from that, and to just you know to, I'd
almost encourage people to be a bit reflective about what is it about what I do that someone else
would want to benefit from. But also, what do I benefit? How do I benefit from working more
openly?
Chris Tibbs:
That's really, really, really good advice. Thank you very much for sharing your knowledge and
experience and hopefully we can inspire some of our listeners to start thinking about, you know,
managing and sharing their data. Thank you everyone for listening and thank you Eilis. Take care.
Bye bye.
Comments (0)
To leave or reply to comments, please download free Podbean or
No Comments
To leave or reply to comments,
please download free Podbean App.